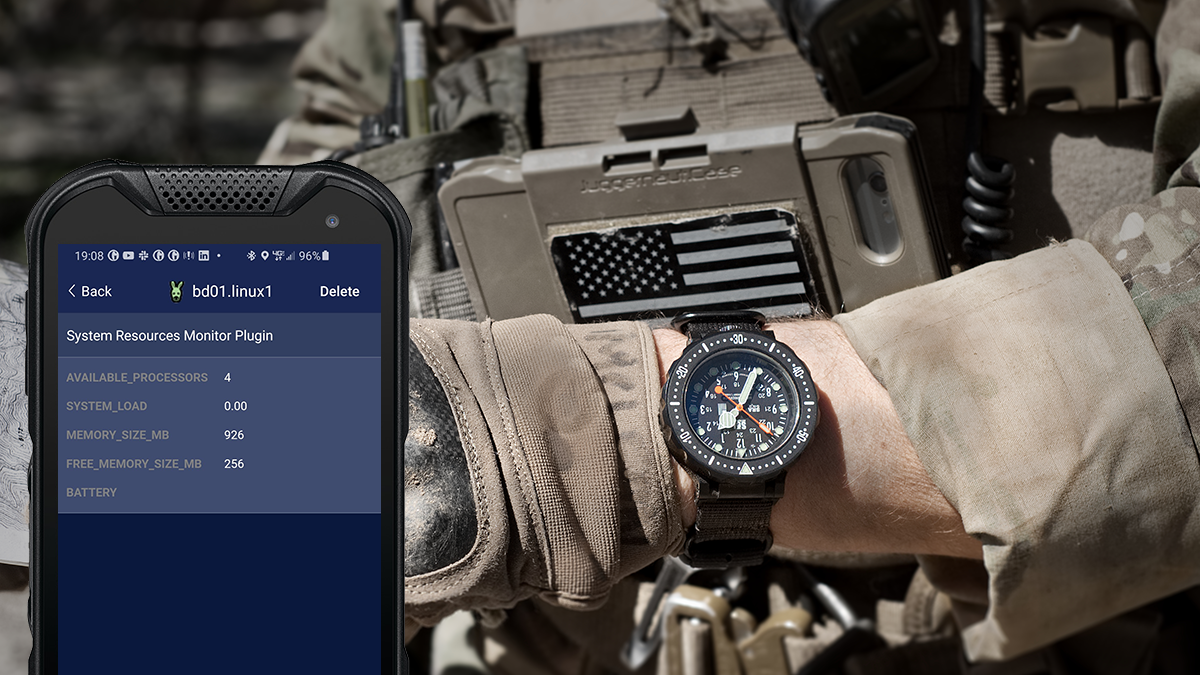
Blueforce adds Recognitional Support for Edge Operations
Blueforce’s new EDGEbox for Linux will Ship With Recognitional Plugins
Given the tempo of operations and the collapsed decision space presented by increasingly non-predictive threats, we continue to hear a common refrain: decision makers want nothing to do with a big data problem when on target, or at an incident site. The “Commander†is too often drowning in data, yet desires an explicit collision of “sense-making†with tactical data, where and when it meets the commander’s intent.
The introduction of the hybrid cloud, where edge-based operations fuse with cloud services, has only recently been realized and Blueforce is proud to be one of the first solution providers to push discrete and on-demand AI to the edge, fused with near cloud and big cloud AI/cognitive services. Combining rapid swarming interfaces and immediate connectivity amongst the right people, sensors, and AI-enabled information services, can enable responders and warfighters to more quickly make sense of what is going on at a scene, without the information overload.
Blueforce’s patented “Decentralized Fusion Engine” (DFE) began with the use of Blueforce software plugins to eliminate edge IoT/sensor silos where the cognitive lift of flipping between 20 Windows apps and/or web apps to make sense of what was going on destroyed the operational value of the data and forced the warfighter or responder to “connect the dots”. When we first shipped, plugins were largely used to pre-process sensor data on the endpoint, and the Blueforce DFE would fuse multiple plugin outputs into a single pre-processed cryptolope of data, and then self-synchronize with other human/sensor endpoints allowed to receive the processed data. It delivered on the promise of the coveted “single pane of glass”, and that was in 2012. While Blueforce plugins have always supported business logic based on rules and thresholds, we have since evolved the DFE to support plugins which encapsulate services that can function at the edge of the network without the need for backhaul. As important, our Inter-Process Messaging enabled plugins to talk to other plugins at bus speeds.
Core to moving “services” to the edge is “recognitional support”, where we pivot from the mere movement of edge-processed data, to detecting and applying meaning across the deployed team, and surface critical and actionable insights across a mosaic of people, sensors, and services which is timely and relevant. Processed recognitional information tells a story of what is going on and can convey “signatures†that denote deviations from expected outcomes, but also surface real-time deviations that signal that things might be going sideways. Some deployed and operational examples of “recognitional” support include:
- Signal: While “rules” might detect a discrete signature of interest, “recognition” can alert that a quantity of unexpected and/or unknown signals are nearby, and are proximate to a location of interest and/or members of the team.
- Clusters: While “rules” may alert on numbers inside of a geofence, “recognition” can flag clusters based on loiter, direction of travel, and/or elevated environmental readings.
- Variations: While “rules” will spot greater-than or less-than deviations in data, “recognition” looks across a corpus of edge sensors and their data, and spots patterns of deviation across the different data sets, across the entire deployed force (human AND silicon).
- Competencies and Resources: While “rules” might tell you what is needed, “recognition” monitors the mission, and can advise when humans with specific competencies and/or resources are available and proximate.
Recognitional COMPUTE is one of our favorites and critical for edge operations where stream analytics and cognitive support are needed (without the need for backhaul). As shared in a prior blog post, Blueforce’s Situational Awareness and IoT edge compute platform for Linux is nearing beta testing and one of the first Blueforce plugins is a System Resource plugin which monitors available compute power and comms on an individual endpoint and creates a floating average of system load, which is then shared amongst approved endpoints at the edge via our Extended Presence Protocol (among other high value metrics to be announced soon). This delivers multiple benefits:
- It enables Blueforce plugins on a local edge device to query it’s own System Resource “load” and decide how and when to execute actions.
- It informs the deployed cadre of edge compute devices of “what” processing is available amongst a myriad of processors, memory, and connectivity channels on human and autonomous endpoints.
- Based on this known available edge endpoint compute capability within the cadre allows for compute handoff amongst subscribed devices when a single node is taxed, or does not have the organic processing capability to executes specific action.
Blueforce is building an entire set of composable services around Recognitional Support specific to single endpoints, but also across an entire collection of endpoints at the edge. More on these in a future post. For more information, visit us at www.blueforcedev.com or Request a Demo.